
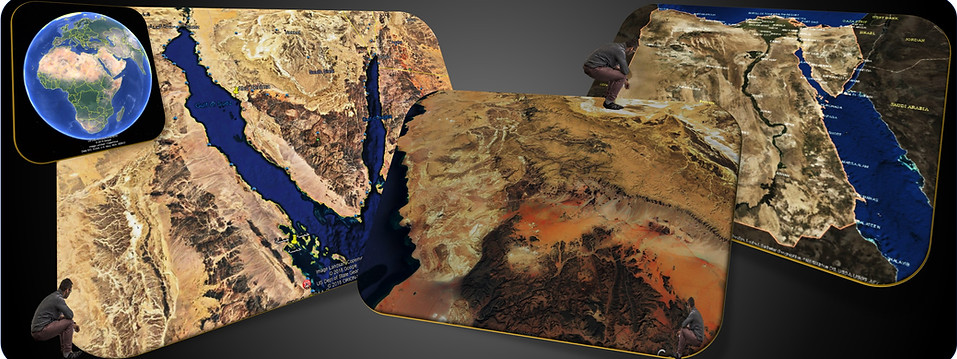

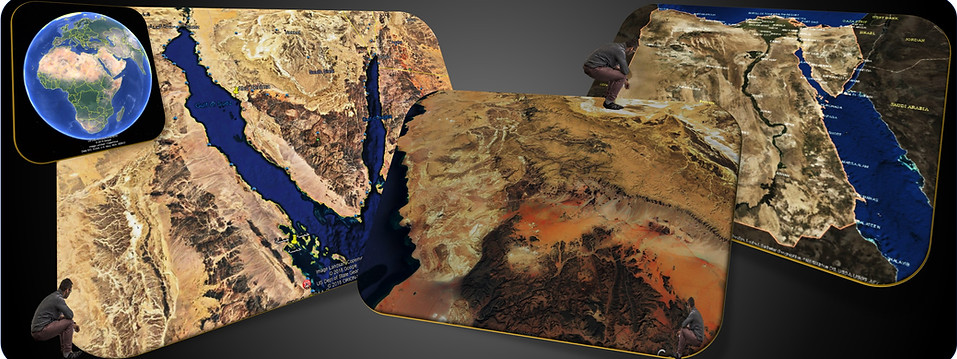


HCS
GEOSCIENCE & ENGINEERING
CONSULTING SERVICES
Reservoir Characterization
Reservoir Characterization is the process of creating an interdisciplinary high-resolution geosciences model that incorporates, integrates, and reconciles various types of geological, geophysical and engineering information from pore to basin scales. They consider maintaining high displacement efficiency, optimizing high sweep efficiency, providing reliable reservoir performance predictions as well as reducing risk and maximizing profits as the main goals of reservoir characterization (Schatzinger and Jordan (1999).
HCS offers a team of experienced geo-scientists and engineers to properly integrate the geological, geophysical, petrophysical, and engineering information consistently, to achieve sustainable modeling project.
​​
Our Reservoir Characterization team has the experience to build best fit models that will be useful for both day to day field monitoring and long-term field development planning.
Petrophysics – Understanding well log measurements and properly calibrating all available data is a critical step in characterizing a reservoir. Our experienced petrophysical staff use cutting edge technology to analyze and condition well logs for optimal characterization results.
Seismic Interpretation and Attribute Analysis provide our clients with the most accurate interpretations and attribute analysis. Seismic attributes are important for understanding rock characteristics away from the well bore, and properly interpreted volumes are necessary to define faults and other geologic structures.
​​
Machine Learning Solutions
​
Using Machine Learning (ML) or Deep Learning (DL) we are able to integrate attributes derived from seismic segy with well logs to build data driven models that predict pseudo logs at every seismic trace. This results in segy volumes representing log values and should be of interest to geologists, petrophysicists and geophysicists. Traditional interpretation workstations can be used to interpret the resulting volumes allowing the detailed mapping of facies and fluids. This is achieved by generating pseudo log segy volumes of Gamma Ray, Resistivity, Neutron and Density.
Besides traditionally extracted post stack attributes, we also use attributes from Empirical Mode Decomposition (EEMD) technology and Topological Data Analysis (TDA) to build ML/DL models. The approach should be beneficial to exploration areas, with a small number of wells, and to development areas with lots of well data.
ML/DL can also be helpful in building Mechanical Earth Models (MEM of Geomechanics) by generating full segy volumes of log derived Poisson's ratio and Young's modulus.
We follow the standard ML workflow of applying train/validate/test splits and cross validation.
​
Geo-modeling a key tool for understanding reservoir behavior is the geologic model.
The static model is manipulated through many possible iterations, giving customers a view of a range of reservoir scenarios. Scenario results are then statistically analyzed to focus on the most probable reservoir conditions.

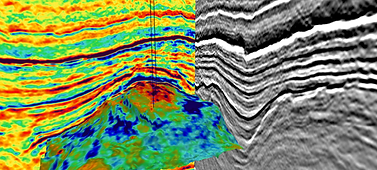


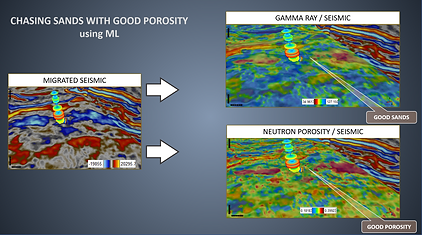

